Webinar Online
Image
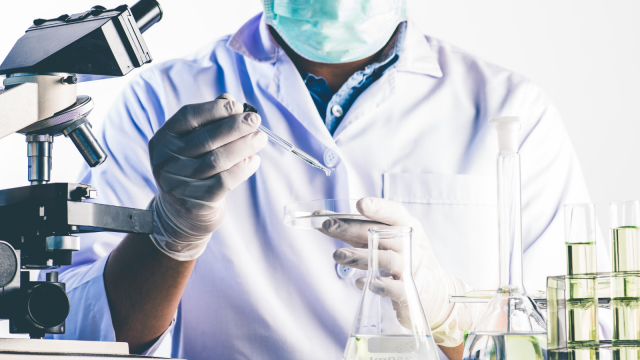
Mercoledì, 12 Maggio 2021 Ore 15
Meeting link https://unimib.webex.com/unimib/j.php?MTID=m8757a1cedd12d253b384fc7100c888b7
Meeting number (access code): 121 481 2534
Meeting password: bnTJ8ttPK48 (26858887 from phones)
Clinical workflows in oncology rely on predictive and prognostic molecular biomarkers. However, the growing number of these complex biomarkers tends to increase the cost and time for decision-making in routine daily oncology practice; furthermore, biomarkers often require tumour tissue on top of routine diagnostic material. Nevertheless, routinely available tumour tissue contains an abundance of clinically relevant information that is currently not fully exploited. Advances in deep learning (DL), an artificial intelligence (AI) technology, have enabled the extraction of previously hidden information directly from routine histology images of cancer, providing potentially clinically useful information. In gastrointestinal and liver cancer, such algorithms can predict survival and molecular alterations. Once pathology workflows are widely digitized, these methods could be used as inexpensive biomarkers. However, clinical translation requires training interdisciplinary researchers in both programming and clinical applications.
Dr Jakob Nicholas Kather Department of Gastroenterology, University Hospital RWTH Aachen, Aachen, Germany
Ospite dei Prof Pietro Invernizzi, Dott. Marco Carbone
Document
Argomento